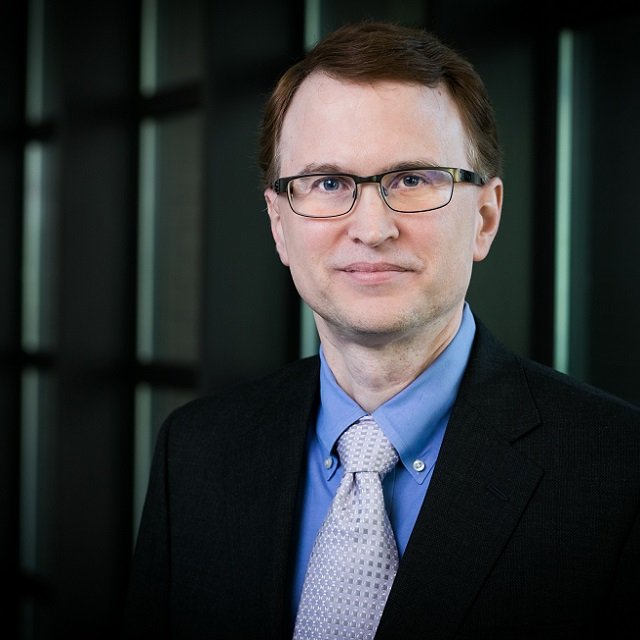
© 2025 ALM Global, LLC, All Rights Reserved. Request academic re-use from www.copyright.com. All other uses, submit a request to [email protected]. For more information visit Asset & Logo Licensing.
Keep up with the latest tax rules and regulations with weekly, exclusive updates by our Tax Facts experts.
Get More Information