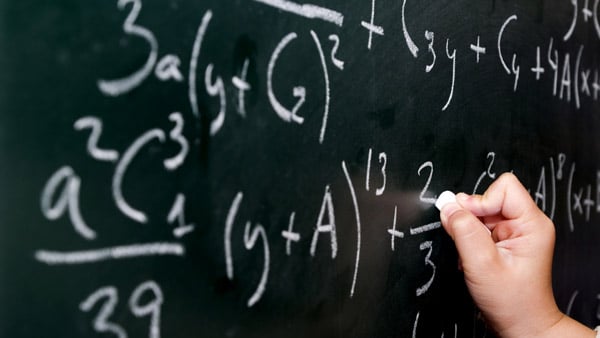
Like alchemists seeking to transform base metals into gold, asset managers are constantly seeking the perfect formula for investment success. In recent years, the search has focused on proprietary quantitative strategies, involving rule-based investments. The genre is ill-defined and marketed under different names, including factor investing, risk parity, smart beta and so forth.
Whatever the rubric, "quant" funds now have over $1.5 trillion under management. Index and quantitative investing account for over half of all equity trading, double the level a decade ago. But, after initial success, they've produced uneven returns recently — a bad run that may well continue. Arguably, the inherent weaknesses of the approach are now being exposed.
Fancy math can't mask the fact that quantitative investing strategies essentially rely on pattern recognition: Models look to correlate past periods of superior returns with specific factors including value, size, volatility, yield, quality and momentum. Once the latter are identified, fund managers construct portfolios with specified return and risk parameters consisting of securities that match those optimal characteristics. Other techniques exploit short-term dislocations between individual prices and comparable securities or the broader market, betting that the relationship will eventually revert to normal.
Such approaches have several fundamental weaknesses. First of all, quant investing is tainted by hindsight bias — the belief that understanding the past allows the future to be predicted. Given enough time, money and computing power, a strategy predicting high returns can be found and validated using back testing to check its historical performance. But, this heightens the risk of overfitting, or adjusting the model to suit a specific set of historical conditions. Those may look like a winning recipe, but could turn out to be an historical fluke.
Modelling is also affected by practical matters, such as what data is or isn't available. London Business School researchers found over 300 factors that could be used to develop potential strategies, heightening the risk of an overfitted model. There is in addition the problem of ergodicity, that is, the lack of a truly representative data sample.
It's important to remember, too, that financial eras are characterized by specific policies, market structures, instruments and investors. Unique conditions that shape returns, volatility and correlation may change. While models create an illusion of sophisticated certainty, they can't capture the full range of events that produced a particular outcome and could perform poorly where a paradigm shift occurs. Modern markets may simply be too complex to be modeled accurately.